报告题目:Penalized empirical likelihood for high-dimensional generalized linear models
报 告 人:陈夏 教授 陕西师范大学
照 片:
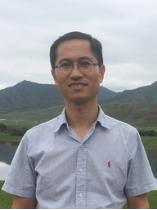
报告时间:2020年11月5号15:00—17:00
报告地点:西电长安校区信远二区205会议室
邀 请 人:冶继民 教授
个人简介:陈夏,陕西师范大学数学与信息科学学院教授、副经理。武汉大学概率论与数理统计专业博士,北京师范大学博士后。兼任陕西省统计学学会副理事长、中国现场统计研究会大数据统计分会理事。主要研究兴趣为广义线性模型、经验似然、高维数据分析和概率极限理论。目前已发表论文20余篇,在科学出版社出版专著1部。主持完成国家自然科学基金2项和陕西省自然科学基金1项。目前主持国家自然科学基金重点项目子课题、教育部人文社科研究项目和陕西省自然科学基金各1项。
报告摘要:We develop penalized empirical likelihood for parameter estimation and variable selection in high-dimensional generalized linear models. By using adaptive lasso penalty function, we show that the proposed estimator has the oracle property. Also, we consider the problem of testing hypothesis, and show that the nonparametric profiled empirical likelihood ratio statistic has asymptotic chi-square distribution. Some simulations and an application are given to illustrate the performance of the proposed method.