报告题目: 机器学习系列报告(三)之神经网络在遥感图像分析中的应用
Procedural Synthesis of Remote Sensing Images for Robust Change Detection with Neural Networks
报告人:Evgeny Burnaev,副教授,俄罗斯斯科尔科沃科技研究院
照片:
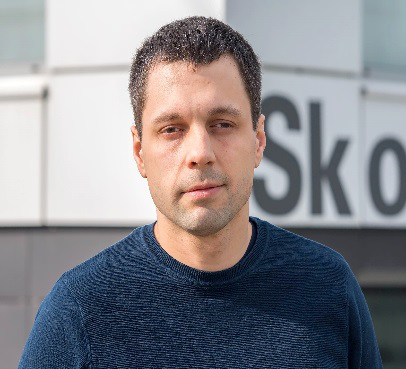
邀请人:董从造
报告时间:2019年05月29日(周三)下午15:00-16:00
报告地点:信远楼II205williamhill威廉希尔官网报告厅
报告人简介:
Evgeny Burnaev obtained his MSc in Applied Physics and Mathematics from the Moscow Institute of Physics and Technology in 2006. After successfully defending his PhD thesis in Foundations of Computer Science at the Institute for Information Transmission Problem RAS in 2008, Evgeny stayed with the Institute as a head of IITP Data Analysis and Predictive Modeling Lab. Since 2007 Evgeny Burnaev carried out a number of successful industrial projects with Airbus, SAFT, IHI, and Sahara Force India Formula 1 team among others as a Head of Data Analysis group in Datadvance llc. Since 2016 Evgeny Burnaev is an Associate Professor of Skoltech and manages his research group for Advanced Data Analytics in Science and Engineering, specialized in industrial analytics and development of machine learning methods. The main research directions are predictive modeling for optimization of technological processes and 3D computer vision with medical applications.
In 2017 Evgeny Burnaev (jointly with Alexey Zaytsev and Maxim Panov) was honored with the Moscow Government Prize for Young Scientists (莫斯科政府青年学者奖,总共四个人获此殊荣) in the category "Transmission, Storage, Processing and Protection of Information" for his scientific contribution and leading the project "The development of methods for predictive analytics for processing industrial, biomedical and financial data."
注:1. Burnaev教授将在公司举行机器学习的系列报告,欢迎参加。
2. Burnaev教授目前正在公司为大三员工和研究生讲授《贝叶斯机器学习》课程,共32学时,前16学时已于3月11日至3月22日讲授完,目前正完成后半部分。欢迎感兴趣的师生前往学习交流。
3. Burnaev教授所在斯科尔科沃科技研究院与MIT联合举办2019机器学习暑期学校,目前开放员工和老师注册,具体信息详见:
https://www.skoltech.ru/en/2019/03/machine-learning-summer-school-2019-at-skoltech/
http://mlss2019.skoltech.ru
https://facebook.com/SkoltechMLSS/
报告摘要:
Abstract:
Data-driven methods such as convolutional neural networks (CNNs) are known to deliver state-of-the-art performance on image recognition tasks when the training data are abundant. However, in some instances, such as change detection in remote sensing images, annotated data cannot be obtained in sufficient quantities. In this work, we propose a simple and efficient method for creating realistic targeted synthetic datasets in the remote sensing domain, leveraging the opportunities offered by game development engines. We provide a description of the pipeline for procedural geometry generation and rendering as well as an evaluation of the efficiency of produced datasets in a change detection scenario. Our evaluations demonstrate that our pipeline helps to improve the performance and convergence of deep learning models when the amount of real-world data is severely limited.
主办单位:williamhill威廉希尔官网